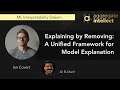
Explaining by Removing: A Unified Framework for Model Explanation | AISC
Speaker(s): Ian Covert
Host(s): Ali Al-Sherif
Find the recording, slides, and more info at https://ai.science/e/explaining-by-removing-a-unified-framework-for-model-explanation--ao4ZaboI76dQLPhX40Bg
Motivation / Abstract
This work highlights the common patterns in 20+ different ML explanation methods including several of the most widely used approaches (SHAP, LIME, Meaningful Perturbations, permutation tests). If there was one paper, you SHOULD read to better understand the choices and assumptions made by many current explanatory methods, it is this one.
------
Aggregate Intellect hosts 3-5 live sessions like this on various AI research, engineering, and product topics every week! Visit https://ai.science for more details
#machinelearning #deeplearning #modelexplainability #explainableai #ai