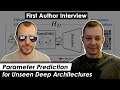
Parameter Prediction for Unseen Deep Architectures (w/ First Author Boris Knyazev)
#deeplearning #neuralarchitecturesearch #metalearning
Deep Neural Networks are usually trained from a given parameter initialization using SGD until convergence at a local optimum. This paper goes a different route: Given a novel network architecture for a known dataset, can we predict the final network parameters without ever training them? The authors build a Graph-Hypernetwork and train on a novel dataset of various DNN-architectures to predict high-performing weights. The results show that not only can the GHN predict weights with non-trivial performance, but it can also generalize beyond the distribution of training architectures to predict weights for networks that are much larger, deeper, or wider than ever seen in training.
OUTLINE:
0:00 - Intro & Overview
6:20 - DeepNets-1M Dataset
13:25 - How to train the Hypernetwork
17:30 - Recap on Graph Neural Networks
23:40 - Message Passing mirrors forward and backward propagation
25:20 - How to deal with different output shapes
28:45 - Differentiable Normalization
30:20 - Virtual Residual Edges
34:40 - Meta-Batching
37:00 - Experimental Results
42:00 - Fine-Tuning experiments
45:25 - Public reception of the paper
ERRATA:
- Boris' name is obviously Boris, not Bori
- At 36:05, Boris mentions that they train the first variant, yet on closer examination, we decided it's more like the second
Paper: https://arxiv.org/abs/2110.13100
Code: https://github.com/facebookresearch/ppuda
Abstract:
Deep learning has been successful in automating the design of features in machine learning pipelines. However, the algorithms optimizing neural network parameters remain largely hand-designed and computationally inefficient. We study if we can use deep learning to directly predict these parameters by exploiting the past knowledge of training other networks. We introduce a large-scale dataset of diverse computational graphs of neural architectures - DeepNets-1M - and use it to explore parameter prediction on CIFAR-10 and ImageNet. By leveraging advances in graph neural networks, we propose a hypernetwork that can predict performant parameters in a single forward pass taking a fraction of a second, even on a CPU. The proposed model achieves surprisingly good performance on unseen and diverse networks. For example, it is able to predict all 24 million parameters of a ResNet-50 achieving a 60% accuracy on CIFAR-10. On ImageNet, top-5 accuracy of some of our networks approaches 50%. Our task along with the model and results can potentially lead to a new, more computationally efficient paradigm of training networks. Our model also learns a strong representation of neural architectures enabling their analysis.
Authors: Boris Knyazev, Michal Drozdzal, Graham W. Taylor, Adriana Romero-Soriano
Links:
TabNine Code Completion (Referral): http://bit.ly/tabnine-yannick
YouTube: https://www.youtube.com/c/yannickilcher
Twitter: https://twitter.com/ykilcher
Discord: https://discord.gg/4H8xxDF
BitChute: https://www.bitchute.com/channel/yannic-kilcher
LinkedIn: https://www.linkedin.com/in/ykilcher
BiliBili: https://space.bilibili.com/2017636191
If you want to support me, the best thing to do is to share out the content :)
If you want to support me financially (completely optional and voluntary, but a lot of people have asked for this):
SubscribeStar: https://www.subscribestar.com/yannickilcher
Patreon: https://www.patreon.com/yannickilcher
Bitcoin (BTC): bc1q49lsw3q325tr58ygf8sudx2dqfguclvngvy2cq
Ethereum (ETH): 0x7ad3513E3B8f66799f507Aa7874b1B0eBC7F85e2
Litecoin (LTC): LQW2TRyKYetVC8WjFkhpPhtpbDM4Vw7r9m
Monero (XMR): 4ACL8AGrEo5hAir8A9CeVrW8pEauWvnp1WnSDZxW7tziCDLhZAGsgzhRQABDnFy8yuM9fWJDviJPHKRjV4FWt19CJZN9D4n