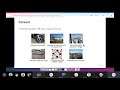
ALIGN: Scaling Up Visual and Vision-Language Representation Learning With Noisy Text Supervision
Pre-trained representations are becoming crucial for many NLP and perception tasks. While representation learning in NLP has transitioned to training on raw text without human annotations, visual and vision-language representations still rely heavily on curated training datasets that are expensive or require expert knowledge. For vision applications, representations are mostly learned using datasets with explicit class labels such as ImageNet or OpenImages. For vision-language, popular datasets like Conceptual Captions, MSCOCO, or CLIP all involve a non-trivial data collection (and cleaning) process. This costly curation process limits the size of datasets and hence hinders the scaling of trained models. In this paper, we leverage a noisy dataset of over one billion image alt-text pairs, obtained without expensive filtering or post-processing steps in the Conceptual Captions dataset. A simple dual-encoder architecture learns to align visual and language representations of the image and text pairs using a contrastive loss. We show that the scale of our corpus can make up for its noise and leads to state-of-the-art representations even with such a simple learning scheme. Our visual representation achieves strong performance when transferred to classification tasks such as ImageNet and VTAB. The aligned visual and language representations enables zero-shot image classification and also set new state-of-the-art results on Flickr30K and MSCOCO image-text retrieval benchmarks, even when compared with more sophisticated cross-attention models. The representations also enable cross-modality search with complex text and text + image queries.
Speaker: Yinfei Yang, Google
Microsoft Research Deep Learning team: https://www.microsoft.com/en-us/research/group/deep-learning-group/