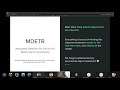
MDETR: Modulated Detection for End-to-End Multi-Modal Understanding
Multi-modal reasoning systems rely on a pre-trained object detector to extract regions of interest from the image. However, this crucial module is typically used as a black box, trained independently of the downstream task and on a fixed vocabulary of objects and attributes. This makes it challenging for such systems to capture the long tail of visual concepts expressed in free form text. In this paper we propose MDETR, an end-to-end modulated detector that detects objects in an image conditioned on a raw text query, like a caption or a question. We use a transformer-based architecture to reason jointly over text and image by fusing the two modalities at an early stage of the model. We pre-train the network on 1.3M text-image pairs, mined from pre-existing multi-modal datasets having explicit alignment between phrases in text and objects in the image. We then fine-tune on several downstream tasks such as phrase grounding, referring expression comprehension and segmentation, achieving state-of-the-art results on popular benchmarks. We also investigate the utility of our model as an object detector on a given label set when fine-tuned in a few-shot setting. We show that our pre-training approach provides a way to handle the long tail of object categories which have very few labelled instances. Our approach can be easily extended for visual question answering, achieving competitive performance on GQA and CLEVR.
Speaker: Aishwarya Kamath, New York University's Center for Data Science
Microsoft Research Deep Learning team: https://www.microsoft.com/en-us/research/group/deep-learning-group/