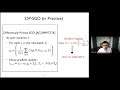
A Geometric View on Private Gradient-Based Optimization
A Google TechTalk, presented by Steven Wu, 2021/04/16
ABSTRACT: Differential Privacy for ML Series. Deep learning models are increasingly popular in many machine learning applications where the training data may contain sensitive information. To provide formal and rigorous privacy guarantees, many learning systems now incorporate differential privacy in their model training. I will talk about our recent studies on the gradient distributions in private training trajectories, which often exhibit low-dimensional and symmetric structures. I will present how our results leverage these geometric structures to derive more accurate training methods and characterize their convergence behaviors.
About the speaker: Steven Wu is an Assistant Professor in the School of Computer Science at Carnegie Mellon University. His research focuses on (1) how to make machine learning better aligned with societal values, especially privacy and fairness, and (2) how to make machine learning more reliable and robust when algorithms interact with social and economic dynamics. In 2017, he received his Ph.D. in computer science at the University of Pennsylvania, where his doctoral dissertation received Penn’s Morris and Dorothy Rubinoff Award for best thesis. His research is supported by an Amazon Research Award, a Facebook Research Award, a Mozilla research grant, a Google Faculty Research Award, a J.P. Morgan Research Faculty Award, and the National Science Foundation.