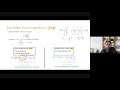
Fast and Memory Efficient Differentially Private-SGD via JL Projections
A Google TechTalk, presented by Sivakanth Gopi, 2021/05/21
ABSTRACT: Differential Privacy for ML Series. Differentially Private-SGD (DP-SGD) of Abadi et al. (2016) and its variations are the only known algorithms for private training of large scale neural networks. This algorithm requires computation of per-sample gradients norms which is extremely slow and memory intensive in practice. In this paper, we present a new framework to design differentially private optimizers called DP-SGD-JL and DP-Adam-JL. Our approach uses Johnson-Lindenstrauss (JL) projections to quickly approximate the per-sample gradient norms without exactly computing them, thus making the training time and memory requirements of our optimizers closer to that of their non-DP versions.
Unlike previous attempts to make DP-SGD faster which work only on a subset of network architectures, we propose an algorithmic solution which works for any network in a black-box manner which is the main contribution of this paper.
About the speaker: Sivakanth Gopi is a senior researcher in the Algorithms group at Microsoft Research Redmond. He is broadly interested in Theoretical Computer Science with a special focus on Coding Theory and Differential Privacy. He is part of the DNA Storage Project, Project Laplace (differential privacy) and Coding for Distributed Storage at Microsoft. He got his PhD from Princeton University in 2018. Before that, he graduated from IIT Bombay with a major in computer science and a minor in mathematics.