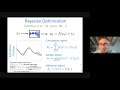
Efficient Exploration in Bayesian Optimization – Optimism and Beyond by Andreas Krause
A Google TechTalk, presented by Andreas Krause, 2021/06/07
ABSTRACT: A central challenge in Bayesian Optimization and related tasks is the exploration—exploitation dilemma: Selecting inputs that are informative about the unknown function, while focusing exploration where we expect high return. In this talk, I will present several approaches based on nonparametric confidence bounds that are designed to navigate this dilemma. We’ll explore the limits of the well-established optimistic principle, especially when we need to distinguish epistemic and aleatoric uncertainty, or in light of constraints. I will also present recent results aiming to meta-learn well-calibrated probabilistic models (Gaussian processes and Bayesian neural networks) from related tasks. I will demonstrate our algorithms on several optimal experimental design tasks.
About the speaker: Andreas Krause is a Professor of Computer Science at ETH Zurich, where he leads the Learning & Adaptive Systems Group. He also serves as Academic Co-Director of the Swiss Data Science Center and Chair of the ETH AI Center, and co-founded the ETH spin-off LatticeFlow. Before that he was an Assistant Professor of Computer Science at Caltech. He received his Ph.D. in Computer Science from Carnegie Mellon University (2008) and his Diplom in Computer Science and Mathematics from the Technical University of Munich, Germany (2004). He is a Microsoft Research Faculty Fellow and a Kavli Frontiers Fellow of the US National Academy of Sciences. He received ERC Starting Investigator and ERC Consolidator grants, the Deutscher Mustererkennungspreis, an NSF CAREER award as well as the ETH Golden Owl teaching award. His research has received awards at several premier conferences and journals, including the ACM SIGKDD Test of Time award 2019 and the ICML Test of Time award 2020. Andreas Krause served as Program Co-Chair for ICML 2018, and is regularly serving as Area Chair or Senior Program Committee member for ICML, NeurIPS, AAAI and IJCAI, and as Action Editor for the Journal of Machine Learning Research.