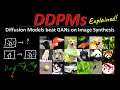
DDPM - Diffusion Models Beat GANs on Image Synthesis (Machine Learning Research Paper Explained)
#ddpm #diffusionmodels #openai
GANs have dominated the image generation space for the majority of the last decade. This paper shows for the first time, how a non-GAN model, a DDPM, can be improved to overtake GANs at standard evaluation metrics for image generation. The produced samples look amazing and other than GANs, the new model has a formal probabilistic foundation. Is there a future for GANs or are Diffusion Models going to overtake them for good?
OUTLINE:
0:00 - Intro & Overview
4:10 - Denoising Diffusion Probabilistic Models
11:30 - Formal derivation of the training loss
23:00 - Training in practice
27:55 - Learning the covariance
31:25 - Improving the noise schedule
33:35 - Reducing the loss gradient noise
40:35 - Classifier guidance
52:50 - Experimental Results
Paper (this): https://arxiv.org/abs/2105.05233
Paper (previous): https://arxiv.org/abs/2102.09672
Code: https://github.com/openai/guided-diffusion
Abstract:
We show that diffusion models can achieve image sample quality superior to the current state-of-the-art generative models. We achieve this on unconditional image synthesis by finding a better architecture through a series of ablations. For conditional image synthesis, we further improve sample quality with classifier guidance: a simple, compute-efficient method for trading off diversity for sample quality using gradients from a classifier. We achieve an FID of 2.97 on ImageNet 128×128, 4.59 on ImageNet 256×256, and 7.72 on ImageNet 512×512, and we match BigGAN-deep even with as few as 25 forward passes per sample, all while maintaining better coverage of the distribution. Finally, we find that classifier guidance combines well with upsampling diffusion models, further improving FID to 3.85 on ImageNet 512×512. We release our code at this https URL
Authors: Alex Nichol, Prafulla Dhariwal
Links:
TabNine Code Completion (Referral): http://bit.ly/tabnine-yannick
YouTube: https://www.youtube.com/c/yannickilcher
Twitter: https://twitter.com/ykilcher
Discord: https://discord.gg/4H8xxDF
BitChute: https://www.bitchute.com/channel/yannic-kilcher
Minds: https://www.minds.com/ykilcher
Parler: https://parler.com/profile/YannicKilcher
LinkedIn: https://www.linkedin.com/in/yannic-kilcher-488534136/
BiliBili: https://space.bilibili.com/1824646584
If you want to support me, the best thing to do is to share out the content :)
If you want to support me financially (completely optional and voluntary, but a lot of people have asked for this):
SubscribeStar: https://www.subscribestar.com/yannickilcher
Patreon: https://www.patreon.com/yannickilcher
Bitcoin (BTC): bc1q49lsw3q325tr58ygf8sudx2dqfguclvngvy2cq
Ethereum (ETH): 0x7ad3513E3B8f66799f507Aa7874b1B0eBC7F85e2
Litecoin (LTC): LQW2TRyKYetVC8WjFkhpPhtpbDM4Vw7r9m
Monero (XMR): 4ACL8AGrEo5hAir8A9CeVrW8pEauWvnp1WnSDZxW7tziCDLhZAGsgzhRQABDnFy8yuM9fWJDviJPHKRjV4FWt19CJZN9D4n