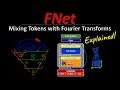
FNet: Mixing Tokens with Fourier Transforms (Machine Learning Research Paper Explained)
#fnet #attention #fourier
Do we even need Attention? FNets completely drop the Attention mechanism in favor of a simple Fourier transform. They perform almost as well as Transformers, while drastically reducing parameter count, as well as compute and memory requirements. This highlights that a good token mixing heuristic could be as valuable as a learned attention matrix.
OUTLINE:
0:00 - Intro & Overview
0:45 - Giving up on Attention
5:00 - FNet Architecture
9:00 - Going deeper into the Fourier Transform
11:20 - The Importance of Mixing
22:20 - Experimental Results
33:00 - Conclusions & Comments
Paper: https://arxiv.org/abs/2105.03824
ADDENDUM:
Of course, I completely forgot to discuss the connection between Fourier transforms and Convolutions, and that this might be interpreted as convolutions with very large kernels.
Abstract:
We show that Transformer encoder architectures can be massively sped up, with limited accuracy costs, by replacing the self-attention sublayers with simple linear transformations that "mix" input tokens. These linear transformations, along with simple nonlinearities in feed-forward layers, are sufficient to model semantic relationships in several text classification tasks. Perhaps most surprisingly, we find that replacing the self-attention sublayer in a Transformer encoder with a standard, unparameterized Fourier Transform achieves 92% of the accuracy of BERT on the GLUE benchmark, but pre-trains and runs up to seven times faster on GPUs and twice as fast on TPUs. The resulting model, which we name FNet, scales very efficiently to long inputs, matching the accuracy of the most accurate "efficient" Transformers on the Long Range Arena benchmark, but training and running faster across all sequence lengths on GPUs and relatively shorter sequence lengths on TPUs. Finally, FNet has a light memory footprint and is particularly efficient at smaller model sizes: for a fixed speed and accuracy budget, small FNet models outperform Transformer counterparts.
Authors: James Lee-Thorp, Joshua Ainslie, Ilya Eckstein, Santiago Ontanon
Links:
TabNine Code Completion (Referral): http://bit.ly/tabnine-yannick
YouTube: https://www.youtube.com/c/yannickilcher
Twitter: https://twitter.com/ykilcher
Discord: https://discord.gg/4H8xxDF
BitChute: https://www.bitchute.com/channel/yannic-kilcher
Minds: https://www.minds.com/ykilcher
Parler: https://parler.com/profile/YannicKilcher
LinkedIn: https://www.linkedin.com/in/yannic-kilcher-488534136/
BiliBili: https://space.bilibili.com/1824646584
If you want to support me, the best thing to do is to share out the content :)
If you want to support me financially (completely optional and voluntary, but a lot of people have asked for this):
SubscribeStar: https://www.subscribestar.com/yannickilcher
Patreon: https://www.patreon.com/yannickilcher
Bitcoin (BTC): bc1q49lsw3q325tr58ygf8sudx2dqfguclvngvy2cq
Ethereum (ETH): 0x7ad3513E3B8f66799f507Aa7874b1B0eBC7F85e2
Litecoin (LTC): LQW2TRyKYetVC8WjFkhpPhtpbDM4Vw7r9m
Monero (XMR): 4ACL8AGrEo5hAir8A9CeVrW8pEauWvnp1WnSDZxW7tziCDLhZAGsgzhRQABDnFy8yuM9fWJDviJPHKRjV4FWt19CJZN9D4n