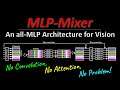
MLP-Mixer: An all-MLP Architecture for Vision (Machine Learning Research Paper Explained)
#mixer #google #imagenet
Convolutional Neural Networks have dominated computer vision for nearly 10 years, and that might finally come to an end. First, Vision Transformers (ViT) have shown remarkable performance, and now even simple MLP-based models reach competitive accuracy, as long as sufficient data is used for pre-training. This paper presents MLP-Mixer, using MLPs in a particular weight-sharing arrangement to achieve a competitive, high-throughput model and it raises some interesting questions about the nature of learning and inductive biases and their interaction with scale for future research.
OUTLINE:
0:00 - Intro & Overview
2:20 - MLP-Mixer Architecture
13:20 - Experimental Results
17:30 - Effects of Scale
24:30 - Learned Weights Visualization
27:25 - Comments & Conclusion
Paper: https://arxiv.org/abs/2105.01601
Abstract:
Convolutional Neural Networks (CNNs) are the go-to model for computer vision. Recently, attention-based networks, such as the Vision Transformer, have also become popular. In this paper we show that while convolutions and attention are both sufficient for good performance, neither of them are necessary. We present MLP-Mixer, an architecture based exclusively on multi-layer perceptrons (MLPs). MLP-Mixer contains two types of layers: one with MLPs applied independently to image patches (i.e. "mixing" the per-location features), and one with MLPs applied across patches (i.e. "mixing" spatial information). When trained on large datasets, or with modern regularization schemes, MLP-Mixer attains competitive scores on image classification benchmarks, with pre-training and inference cost comparable to state-of-the-art models. We hope that these results spark further research beyond the realms of well established CNNs and Transformers.
Authors: Ilya Tolstikhin, Neil Houlsby, Alexander Kolesnikov, Lucas Beyer, Xiaohua Zhai, Thomas Unterthiner, Jessica Yung, Daniel Keysers, Jakob Uszkoreit, Mario Lucic, Alexey Dosovitskiy
ERRATA: Here is their definition of what the 5-shot classifier is: "we report the few-shot accuracies obtained by solving the L2-regularized linear regression problem between the frozen learned representations of images and the labels"
Links:
TabNine Code Completion (Referral): http://bit.ly/tabnine-yannick
YouTube: https://www.youtube.com/c/yannickilcher
Twitter: https://twitter.com/ykilcher
Discord: https://discord.gg/4H8xxDF
BitChute: https://www.bitchute.com/channel/yannic-kilcher
Minds: https://www.minds.com/ykilcher
Parler: https://parler.com/profile/YannicKilcher
LinkedIn: https://www.linkedin.com/in/yannic-kilcher-488534136/
BiliBili: https://space.bilibili.com/1824646584
If you want to support me, the best thing to do is to share out the content :)
If you want to support me financially (completely optional and voluntary, but a lot of people have asked for this):
SubscribeStar: https://www.subscribestar.com/yannickilcher
Patreon: https://www.patreon.com/yannickilcher
Bitcoin (BTC): bc1q49lsw3q325tr58ygf8sudx2dqfguclvngvy2cq
Ethereum (ETH): 0x7ad3513E3B8f66799f507Aa7874b1B0eBC7F85e2
Litecoin (LTC): LQW2TRyKYetVC8WjFkhpPhtpbDM4Vw7r9m
Monero (XMR): 4ACL8AGrEo5hAir8A9CeVrW8pEauWvnp1WnSDZxW7tziCDLhZAGsgzhRQABDnFy8yuM9fWJDviJPHKRjV4FWt19CJZN9D4n