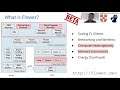
Flower: A Friendly Federated Learning Framework
A Google TechTalk, 2020/7/29, presented by Nicholas Lane, University of Cambridge.
ABSTRACT: Full title: Flower: A Friendly Federated Learning Framework .. and a first look into the carbon footprint of federated methods
Federated Learning (FL) has emerged as a promising technique for edge devices to collaboratively learn a shared prediction model, while keeping their training data on the device, thereby decoupling the ability to do machine learning from the need to store the potentially privacy sensitive user data in the cloud. However, despite rapid the progress made in FL in recent years, it still remains far too difficult to evaluate FL algorithms under a full range of realistic system constraints (viz. compute, memory, energy, wired/wireless networking) and scale (thousands of federated devices and larger). As a consequence, our understanding of how these factors influence FL performance and should shape the future evolution of FL algorithms remains in a very underdeveloped state.
In this talk, I will describe how we have begun to address this situation by developing Flower -- an open-source framework (http://flower.dev) built to help bridge this gap in evaluation and design. Through Flower, it becomes relatively simple to measure the impact of common real-world FL situations, such as if participating devices have limited compute resources (e.g., an embedded device), or when network speeds are highly varied and unstable. I will highlight early empirical observations, made using Flower, as to what the implications are for existing algorithms under the types of heterogeneous large-scale FL systems we anticipate will increasingly appear. Finally, to showcase the potential and flexibility of Flower, I will show how it can even be used to make assessments of the carbon footprint of FL in various settings -- to the best of our knowledge, this is the first time FL has been studied from the perspective of its environmental impact. "